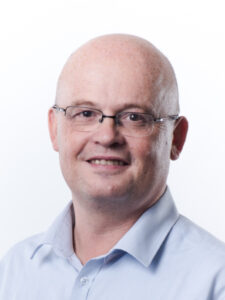
At Amplitude, I faced a persistent challenge: the extensive time required for process development in laser technology. In spite of a global network of application labs, it was difficult to keep up with a growing demand for customer-specific solutions.
From the very beginning, Artifical Intelligence appeared as a key for simpler, faster and more efficient process development. Still, much remains to be done in integrating AI with laser technology.
Starting the journey
At h-nu, our mission is to turn the promise of AI in laser technology into practical, impactful tools to address today’s challenges. Our approach is to start with a down-to-earth, engineering driven vision, and develop smart, AI-based solutions tailored for laser material processing.
To achieve this, we’ve outlined a straightforward, three-step strategy for typical projects:
Step 1: Data Acquisition
The first challenge in applying machine learning to laser processing is data acquisition. The success of many machine learning algorithms depends on the quality and volume of data. However, gathering this data is often slow, and manual labeling is seen as a tedious task by most process engineers.three-step strategy for typical projects:
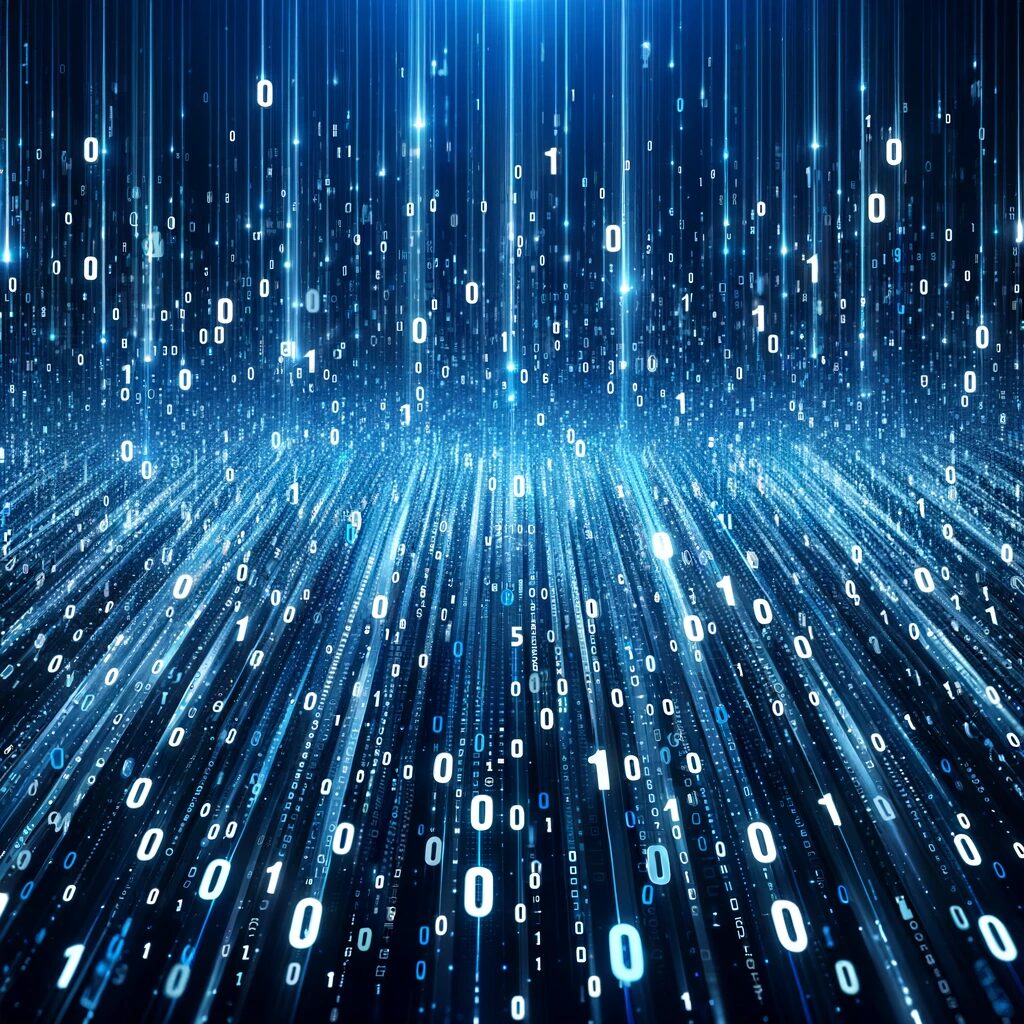
How We Can Help:
- Establish efficient data collection practices, adhering to FAIR (Findability, Accessibility, Interoperability, and Reusability) principles.
- Support manual or automatic data labelling
- Define and implement online diagnostics and automatic data acquisition
Step 2: Model Development
Choosing the correct machine learning model from the many options available is challenging. A deep understanding of the physics and engineering involved is crucial to selecting the most effective approach. This will guide the choice of the most suitable model, whether it be numerical models, machine learning regression, Bayesian optimization, or reinforcement learning.
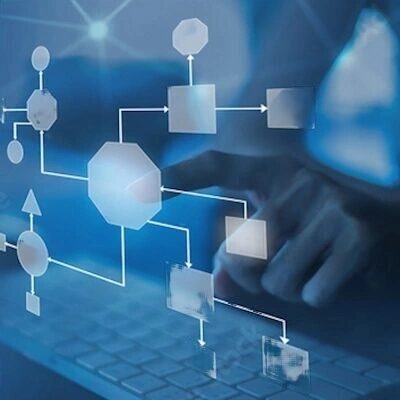
How We Can Help:
- Define use case and requirements
- Guide model selection to ensure functionality and predictability
- Develop and implement numerical or machine learning models.
Step 3: Industrial Implementation
Even after a successful proof of concept, significant work is required for industrial deployment. It is essential to ensure the model performs consistently across various conditions, to establish a monitoring system, and to regularly evaluate prediction accuracy.
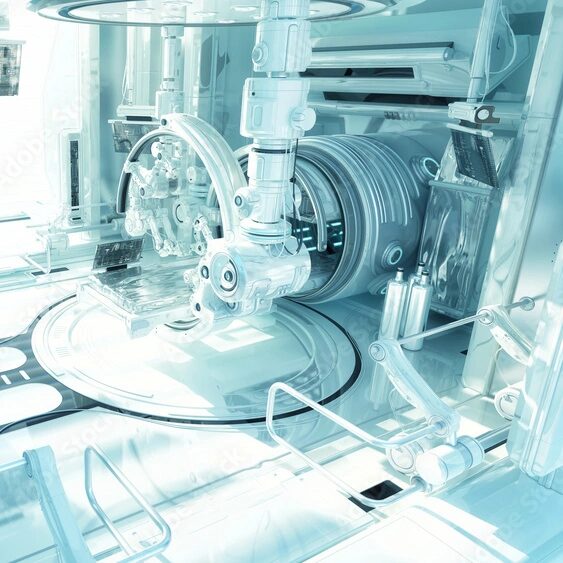
How We Can Help:
- Validate models in diverse environments to ensure broad applicability.
- Define and implement online diagnostic tools for continuous performance monitoring and data validation.
- Ensure scalability to accommodate future growth and increasing data volumes without loss of performance.