ALPhANOV, a leading photonics technology center, specializes in laser applications and process development. In partnership with h-nu, the EMOTIAN project applies machine learning (ML) to laser-based semiconductor failure analysis, specifically improving laser thinning—a key step in accessing integrated circuits (ICs) for diagnostics. By combining ALPhANOV’s expertise in laser processing with h-nu’s ML capabilities, the project aims to make semiconductor failure analysis more precise, reproducible, and automated.
Silicon Thinning for Laser Failure Analysis
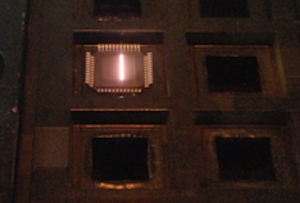
In semiconductor diagnostics, thinning the chip is essential to expose internal circuits for photoemission analysis and other inspection techniques. The process requires precise material removal to avoid damaging the IC while ensuring clear access for investigation.
Traditional laser thinning relies on operator skill and trial-and-error adjustments, leading to variations in depth control and surface quality. The EMOTIAN project integrates ML models to shift from manual tuning to predictive, data-driven control, improving process stability and reproducibility.
Challenges in Data Acquisition
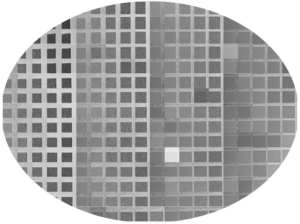
ML models require high-quality datasets, but data acquisition in laser processing is slow and complex. Unlike typical ML applications that use large datasets, semiconductor thinning provides limited experimental data, making efficient ML model training critical.
To address this, the project has automated data collection using a confocal profilometer, ensuring precise and repeatable surface measurements. Other techniques may also be incorporated to improve data acquisition speed and quality, further strengthening ML predictions.
Machine Learning Models for Process Optimization
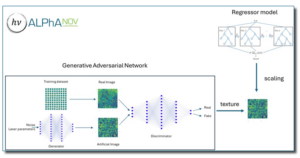
Two key ML approaches are being implemented:
- Regression Models predict thinning depth (d) and surface roughness (Sa) based on laser parameters, ensuring a more controlled and repeatable process.
- GAN (Generative Adversarial Network) + Regression Models analyze and predict surface texture, allowing for finer control over material removal.
These models enhance process accuracy, efficiency, and reproducibility, reducing trial-and-error and enabling faster optimization.
Future Perspectives
As the EMOTIAN project progresses, further automation and real-time process control will be explored. The integration of closed-loop feedback could make laser thinning fully autonomous, reducing variability and increasing efficiency. By combining ML with advanced laser processing, ALPhANOV and h-nu are pioneering a new approach to semiconductor failure analysis, making it faster, more precise, and highly repeatable.